Leveraging Data Analytics to Improve Performance in Behavioral Health RCM
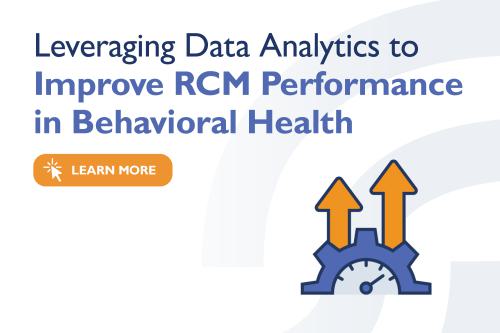
Behavioral health organizations face unique challenges in managing their revenue cycle effectively. As the complexity of billing and reimbursement processes continues to grow, data analytics has emerged as a powerful tool to optimize Revenue Cycle Management (RCM). By harnessing the power of data-driven insights, behavioral health providers can streamline operations, reduce claim denials, and ultimately improve their financial health.
This blog post explores how leveraging data analytics can significantly enhance behavioral health RCM performance. We’ll delve into key performance indicators, implementation strategies, and best practices to help your organization harness the full potential of data analytics in RCM.
Understanding Key Performance Indicators (KPIs) in Behavioral Health RCM
To achieve the positive financial impact of data analytics in behavioral health RCM, it’s crucial to understand and track the right Key Performance Indicators (KPIs). These metrics provide valuable insights into the health of your revenue cycle and highlight areas for improvement. Some essential KPIs for behavioral health RCM include:
- Days in Accounts Receivable (A/R): This metric measures the average time it takes to collect payment after a service is provided. A lower number indicates more efficient collections.
- Denial rates: This KPI tracks the percentage of claims denied by payers. High denial rates can significantly impact cash flow and require additional resources to resolve.
- Collection ratios: This measure compares the amount collected to the amount billed, providing insights into the effectiveness of your billing and collection processes.
- Clean claim rates: This KPI represents the percentage of claims that are accepted and paid on the first submission, without requiring additional information or corrections.
By consistently monitoring these KPIs, organizations can gain a comprehensive view of their behavioral health RCM performance and identify areas for improvement.
Implementing Data Analytics Tools
To effectively leverage data analytics in behavioral health RCM, organizations need to implement robust analytics tools. These tools should be capable of:
- Integrating with existing systems: The analytics solution should seamlessly integrate with your Electronic Health Record (EHR) system and other relevant databases to ensure comprehensive data analysis.
- Providing real-time dashboards and reporting capabilities: Look for tools that offer intuitive, interactive dashboards that make complex data easily understandable and actionable.
- Offering predictive analytics capabilities: Advanced analytics tools should be able to forecast trends and identify potential issues before they impact your revenue cycle.
- Ensuring scalability and flexibility: Choose tools that can grow with your organization and adapt to changing needs, allowing for easy addition of new data sources or analytics capabilities as your needs evolve.
- Considering cost and return on investment: Evaluate the total cost of ownership for analytics tools, including implementation, training, and ongoing support, and weigh this against the expected benefits and efficiency gains.
When selecting an analytics tool, consider solutions specifically designed for behavioral health RCM. These specialized tools often come with pre-built templates and industry-specific insights that can accelerate your analytics journey.
Using Analytics to Streamline Claims Submission and Follow-up
Data analytics can play a crucial role in optimizing the claims submission and follow-up processes. By analyzing historical claims data, organizations can:
- Identify patterns in claim denials: Analytics can reveal common reasons for denials, allowing you to address these issues proactively.
- Optimize claim submission processes: Data-driven insights can help you refine your claims submission workflow, reducing errors and improving clean claim rates.
- Prioritize follow-up efforts: Analytics can help you identify high-value claims or those most likely to be paid, allowing you to allocate resources more effectively in the follow-up process.
Enhancing Denial Management with Data Insights
Effective denial management is critical for maintaining healthy behavioral health RCM. Data analytics can significantly enhance your denial management strategies by:
- Analyzing root causes of denials: Advanced analytics can uncover underlying patterns and trends in denials, helping you address systemic issues.
- Developing targeted strategies for denial prevention: With data-driven insights, you can implement focused interventions to prevent common denial reasons.
- Improving appeal processes: Analytics can help you identify which denied claims are most likely to be overturned on appeal, allowing you to prioritize your appeal efforts.
- Creating a feedback loop for continuous improvement: Establish a system where insights from denial analysis are regularly fed back into the claims submission process, continuously refining and improving your approach.
Optimizing Accounts Receivable Management
Data analytics can transform your approach to accounts receivable management:
- Using predictive analytics for cash flow forecasting: Advanced algorithms can predict future cash flows based on historical data and current trends, enabling better financial planning.
- Identifying high-risk accounts: Analytics can flag accounts that are at risk of non-payment, allowing for early intervention.
- Prioritizing collection efforts: Data-driven insights can help you focus your collection efforts on accounts most likely to yield results, improving overall collection rates.
- Segmenting accounts based on various factors: Use analytics to categorize accounts based on factors like payer type, balance age, or patient demographics, allowing for more targeted collection strategies.
- Automating payment reminders: Implement systems that automatically send payment reminders to patients or payers based on predefined criteria, improving collection rates without increasing staff workload.
Improving Payer Contract Negotiations
Data analytics can provide valuable leverage in payer contract negotiations:
- Analyzing payer performance data: By tracking metrics like average reimbursement rates and denial patterns for each payer, you can identify areas for improvement in your contracts.
- Identifying opportunities for contract improvements: Analytics can reveal specific services or procedures where your reimbursement rates are below market averages, providing concrete data to support your negotiation efforts.
- Supporting negotiations with data-driven insights: Armed with detailed performance data, you can make a compelling case for improved contract terms.
- Benchmarking against industry standards: Use analytics to compare your reimbursement rates and contract terms with industry benchmarks, providing leverage in negotiations and ensuring competitive agreements.
Enhancing Operational Efficiency
Beyond financial metrics, data analytics can drive overall operational efficiency in your behavioral health RCM processes:
- Identifying bottlenecks in RCM processes: Analytics can pinpoint areas where claims or payments are getting stuck, allowing you to streamline your workflows.
- Measuring staff productivity: By analyzing individual and team performance metrics, you can identify top performers and areas where additional training may be needed.
- Informing resource allocation decisions: Data-driven insights can help you determine where to allocate staff and other resources for maximum impact on your revenue cycle.
- Automating routine tasks: Identify repetitive, time-consuming tasks in your RCM workflow and implement automation solutions, freeing up staff time for more complex, high-value activities.
- Implementing predictive modeling for workload management: Use historical data to forecast busy periods or potential bottlenecks, allowing for proactive staffing and resource allocation.
Adapting to Value-Based Care Models
As the healthcare industry shifts towards value-based care, data analytics becomes even more critical:
- Tracking and reporting on quality measures: Analytics tools can help you monitor and report on the quality metrics tied to value-based reimbursement.
- Demonstrating outcomes and value: With comprehensive data analysis, you can clearly demonstrate the value and effectiveness of your services to payers and other stakeholders.
- Aligning RCM processes with value-based payment structures: Analytics can help you adjust your RCM processes to accommodate new payment models and ensure you’re capturing all eligible reimbursements.
Leveraging Predictive Analytics
Predictive analytics represents the cutting edge of data analytics in RCM:
- Forecasting claim denials: Advanced algorithms can predict which claims are likely to be denied, allowing for preemptive action.
- Predicting patient payment behavior: Analytics can help you anticipate which patients are likely to pay their bills on time, informing your collection strategies.
- Anticipating changes in payer policies or reimbursement rates: By analyzing trends and patterns, predictive analytics can help you prepare for potential changes in the reimbursement landscape.
- Identifying trends in patient behavior or treatment outcomes: Analyze patterns in patient data to predict future health needs or treatment responses, enabling more proactive and personalized care delivery.
Challenges in Implementing Data Analytics
While the benefits of data analytics in behavioral health RCM are clear, implementation can come with challenges:
- Data quality and integration issues: Ensuring data accuracy and integrating data from multiple sources can be complex.
- Staff training and adoption: Implementing new analytics tools often requires significant staff training and change management efforts.
- Balancing data-driven decisions with clinical judgment: It’s crucial to use data analytics as a tool to support, not replace, clinical decision-making.
- Managing costs and demonstrating ROI: Carefully track the costs associated with analytics implementation and use, and develop methods to quantify the benefits and demonstrate return on investment to stakeholders.
- Keeping pace with rapidly evolving technology: Stay informed about advancements in analytics technology and be prepared to update or replace systems as needed to maintain competitive advantage.
Best Practices for Successful Analytics Implementation
To maximize the benefits of data analytics in your RCM processes:
- Ensuring data accuracy and completeness: Implement robust data governance practices to maintain high-quality data.
- Providing user-friendly interfaces and visualizations: Choose analytics tools that present data in easily understandable formats.
- Fostering a data-driven culture: Encourage staff at all levels to use data in their decision-making processes.
- Conducting regular staff training and updates: Provide ongoing education to ensure all staff members can effectively use analytics tools and interpret data insights.
- Establishing clear Key Performance Indicators (KPIs): Define specific, measurable metrics that align with your organizational goals to guide your analytics efforts and measure success.
- Performing regular audits of analytics processes: Conduct periodic reviews of your analytics workflows and outputs to ensure accuracy, relevance, and compliance with regulations.
- Collaborating across departments for holistic insights: Foster communication and data sharing between different departments to gain a more comprehensive understanding of your organization’s performance and opportunities for improvement.
- Leveraging specialized behavioral health analytics solutions: Consider implementing analytics platforms specifically designed for behavioral health RCM, which often come with pre-built dashboards, industry-specific KPIs, and insights tailored to the unique challenges of behavioral health organizations. These specialized solutions can significantly accelerate your analytics journey and provide more relevant, actionable insights for your specific operational context.
Leveraging data analytics in RCM can transform the financial performance of behavioral health organizations. By providing deep insights into key performance indicators, streamlining claims processes, enhancing denial management, and supporting strategic decision-making, data analytics empowers behavioral health providers to optimize their revenue cycle and adapt to the changing healthcare landscape.
As the industry continues to evolve, organizations that embrace data-driven approaches to RCM will be best positioned to thrive, ensuring they can continue to provide high-quality care to their patients while maintaining financial stability.
How SimiTree Can Help
Ready to dive deeper into the world of data analytics and RCM in behavioral health? Download our comprehensive eBook, “Mastering Revenue Cycle Management in Behavioral Health,” for in-depth insights and strategies to optimize your RCM performance.
And if you’re looking for powerful, tailored analytics solutions for your behavioral health organization, explore SimiTree’s data analytics capabilities. Our platform offers comprehensive RCM analytics specifically designed for behavioral health providers, helping you turn data into actionable insights that drive financial success.
Don’t let valuable data insights slip through your fingers. Take the first step towards data-driven RCM excellence today!