Overcoming Data Analytics Challenges in Behavioral Health
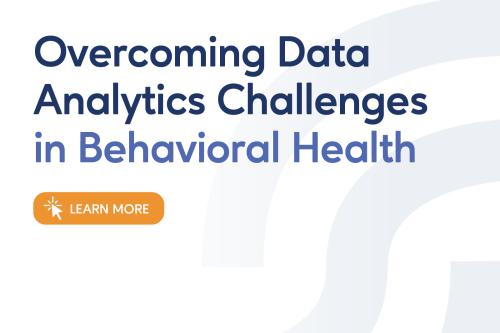
The behavioral health sector is increasingly recognizing the transformative potential of data analytics. From improving patient outcomes to enhancing operational efficiency, the benefits are clear. However, implementing effective data analytics strategies in behavioral health organizations comes with its own set of unique challenges. This article explores these challenges and provides actionable strategies for overcoming them, enabling organizations to harness the full power of their data.
Understanding the Landscape of Data Analytics in Behavioral Health
Before diving into the challenges, it’s essential to understand the critical role data analytics plays in behavioral health. Data analytics empowers behavioral health organizations to:
- Make informed decisions based on real-time insights
- Optimize operations for peak efficiency
- Improve patient outcomes through data-driven care plans
- Gain valuable insights into organizational performance
- Identify areas for improvement and growth
- Develop targeted strategies for long-term success
With these benefits in mind, let’s explore the primary challenges behavioral health organizations face when implementing data analytics and discuss strategies to overcome them.
Behavioral Health Data Analytics Challenges and Solutions
Data Silos and Lack of Interoperability
Challenge:
One of the most significant hurdles in behavioral health data analytics is the existence of data silos. Many organizations use multiple software systems that don’t communicate effectively with each other, leading to:
- Fragmented patient information
- Difficulty in obtaining a comprehensive view of patient care
- Inefficiencies in data collection and analysis
- Potential for errors due to incomplete information
Solution:
Overcoming data silos requires a multi-faceted approach:
- Invest in integration technologies: Implement robust ETL (Extract, Transform, Load) tools that can pull data from various sources into a centralized analytics platform.
- Adopt interoperability standards: Embrace standards like HL7 FHIR to ensure seamless data exchange between different systems.
- Implement a data lake or data warehouse: Create a centralized repository for all your organization’s data, enabling comprehensive analysis across all data sources.
- Prioritize API-first solutions: When selecting new software, prioritize solutions that offer robust APIs for easy integration with other systems.
Data Quality Issues
Challenge:
The accuracy and reliability of analytics depend on the quality of the underlying data. Behavioral health organizations often struggle with:
- Incomplete data: Missing information in patient records or inconsistent data entry practices
- Inaccurate data: Errors in data entry or outdated information
- Inconsistent data: Variations in how data is recorded across different providers or systems
Solution:
Addressing data quality issues requires ongoing effort and attention:
- Implement robust data governance practices: Establish clear policies and procedures for data management, including data ownership, access rights, and quality standards.
- Conduct regular data audits: Systematically review your data for completeness, accuracy, and consistency.
- Use data validation tools: Implement automated checks to flag potential errors or inconsistencies in real time.
- Provide staff training: Ensure all staff understand the importance of data quality and are trained in proper data entry procedures.
- Implement Master Data Management (MDM): Develop a single, authoritative source of truth for key data elements to ensure consistency across systems.
Limited Budgets and Resources
Challenge:
Many behavioral health organizations, especially smaller providers, face resource constraints that can hinder their ability to implement comprehensive data analytics solutions. This includes:
- Limited financial resources for investing in advanced analytics tools
- Insufficient IT infrastructure to support data-intensive operations
- Lack of dedicated staff for data management and analysis
Solution:
Creative solutions can help organizations overcome resource limitations:
- Leverage cloud-based analytics platforms: These often require lower upfront investment and can scale as your needs grow.
- Explore shared services models: Partner with other organizations to share the costs of analytics infrastructure and expertise.
- Prioritize high-impact projects: Start with analytics initiatives that can demonstrate quick wins and ROI to build support for further investment.
- Seek grant funding: Look for grants specifically aimed at improving data capabilities in behavioral health organizations.
- Consider outsourcing: Partner with analytics service providers to access expertise without the need for full-time, in-house staff.
Workforce Skills Gap
Challenge:
The effective use of data analytics requires specialized skills that are often in short supply in the behavioral health workforce. Organizations may struggle with:
- Recruiting data scientists and analysts with healthcare expertise
- Training existing staff on data analytics concepts and tools
- Retaining skilled analytics professionals in a competitive job market
Solution:
Bridging the skills gap requires a multi-pronged approach:
- Invest in training programs: Develop comprehensive training programs to upskill existing staff in data analytics.
- Partner with educational institutions: Collaborate with local colleges and universities to develop programs that combine behavioral health and data analytics skills.
- Implement mentorship programs: Pair data-savvy staff with those looking to develop their skills to foster knowledge sharing.
- Leverage user-friendly analytics tools: Choose analytics platforms with intuitive interfaces that non-technical staff can use effectively.
- Consider hybrid roles: Create positions that combine clinical expertise with data analytics skills to bridge the gap between data and practice.
Privacy and Security Concerns
Challenge:
Health data is highly sensitive, and organizations must navigate complex privacy regulations while leveraging analytics. Challenges include:
- Ensuring compliance with HIPAA and other privacy laws
- Protecting patient data from breaches and unauthorized access
- Balancing data sharing for care coordination with privacy protections
Solution:
Addressing privacy and security concerns is crucial for successful data analytics implementation:
- Implement robust security measures: Use encryption, secure access controls, and regular security audits to protect sensitive data.
- Develop clear data governance policies: Establish guidelines for data use, sharing, and protection that align with regulatory requirements.
- Conduct regular risk assessments: Identify and address potential vulnerabilities in your data management practices.
- Invest in staff training: Ensure all staff understand privacy regulations and best practices for data protection.
- Use data de-identification techniques: Implement methods to anonymize data for analytics purposes while maintaining its utility.
Cultural Resistance and Change Management
Challenge:
Implementing data analytics often requires significant cultural and operational changes within an organization. Resistance can stem from:
- Skepticism about the value of data-driven decision-making
- Concerns about job security or changes in job roles
- Discomfort with new technologies or processes
Solution:
Effective change management is key to overcoming cultural resistance:
- Communicate the vision: Clearly articulate how data analytics will benefit patients, staff, and the organization as a whole.
- Secure leadership buy-in: Ensure that leaders at all levels champion the data-driven approach and model its use.
- Start with pilot projects: Begin with small-scale initiatives to demonstrate success and build momentum.
- Celebrate early wins: Share success stories to reinforce the value of data-driven approaches.
- Provide ongoing support: Offer continuous training and support to help staff adapt to new processes and tools.
Lack of Standardization
Challenge:
The behavioral health field often lacks standardized data collection and reporting practices, making it difficult to:
- Compare data across organizations or regions
- Benchmark performance effectively
- Aggregate data for large-scale analysis
Solution:
Addressing standardization challenges requires both organizational and industry-wide efforts:
- Adopt industry standards: Implement widely recognized standards for data collection and reporting in behavioral health.
- Participate in data-sharing initiatives: Join regional or national data-sharing programs to contribute to and benefit from standardized data sets.
- Engage in industry collaborations: Participate in efforts to develop and promote data standards specific to behavioral health.
- Implement standardized assessment tools: Use validated, standardized assessment instruments to ensure consistency in data collection.
How SimiTree Can Help
SimiTree offers comprehensive solutions to help behavioral health organizations overcome data analytics challenges. Our Afia Navigator platform is designed to address the unique needs of behavioral health providers, offering:
- Seamless data integration from multiple sources
- Advanced data quality management tools
- User-friendly interfaces for non-technical staff
- Robust security features to ensure HIPAA compliance
- Customizable dashboards and reports for different stakeholders
Our team of experts provides end-to-end support, from initial assessment and strategy development to implementation and ongoing optimization. With SimiTree, you gain a partner committed to helping you navigate the complexities of data analytics in behavioral health, ensuring you can harness the full power of your data to improve patient outcomes and organizational performance.
Frequently Asked Questions
What are the main challenges in implementing data analytics in behavioral health?
The main challenges in implementing data analytics in behavioral health include data silos and lack of interoperability, data quality issues, limited budgets, and resources, workforce skills gaps, privacy and security concerns, cultural resistance to change, and lack of standardization in data collection and reporting practices.
How can behavioral health organizations overcome data quality issues?
Behavioral health organizations can overcome data quality issues by implementing robust data governance practices, conducting regular data audits, using data validation tools, providing staff training on proper data entry procedures, and implementing Master Data Management (MDM) to ensure consistency across systems.
What strategies can help address the workforce skills gap in behavioral health data analytics?
Strategies to address the workforce skills gap in behavioral health data analytics include investing in training programs for existing staff, partnering with educational institutions to develop relevant programs, implementing mentorship programs, leveraging user-friendly analytics tools, and creating hybrid roles that combine clinical expertise with data analytics skills.